Cassava is the third-largest food carbohydrate source in the tropics after rice and maize[1][2]. It is also the major staple food in developing countries, especially in sub-Saharan Africa. Accurate assessment and forecasting of cassava yield well before harvest are crucially important for food security and better crop management to ensure sustainable food production systems and implement resilient agricultural practices.
As an emerging technology, unmanned aerial vehicles (UAV) and remote sensing are expected to be useful for cassava yield prediction. We believe that they are potentially important for the acquisition of data to provide spatial and temporal data for site-specific crop management.
Development of Cassava Yield Prediction Model
Recently, Anavision has successfully built a cassava yield prediction model for our business partner by combining Aerial Survey, GIS and Field Survey to capture the following information that may affect cassava production.
- Land Condition
- Land Suitability Analysis (LSA) to classify the land condition grade. The analysis includes Slope, Rainfall, Elevation, Distance from Rivers and Roads, Soil type, Land Cover
- Plant growth
- Information on plant growth can be extracted from regular Aerial Survey data, which is called biophysical Information
- Pests & Disease
- Using multispectral images captured by drones to identify plant health. Aerial images are transformed to generate vegetation indexes: NDVI, NDRE, SAVI, IRECI. All can be used to determine the crop yield
- Plant Information
- Collect plant information such as Planting Date/Age, Seeds, Yield Information, Owner, and Boundary from field owner
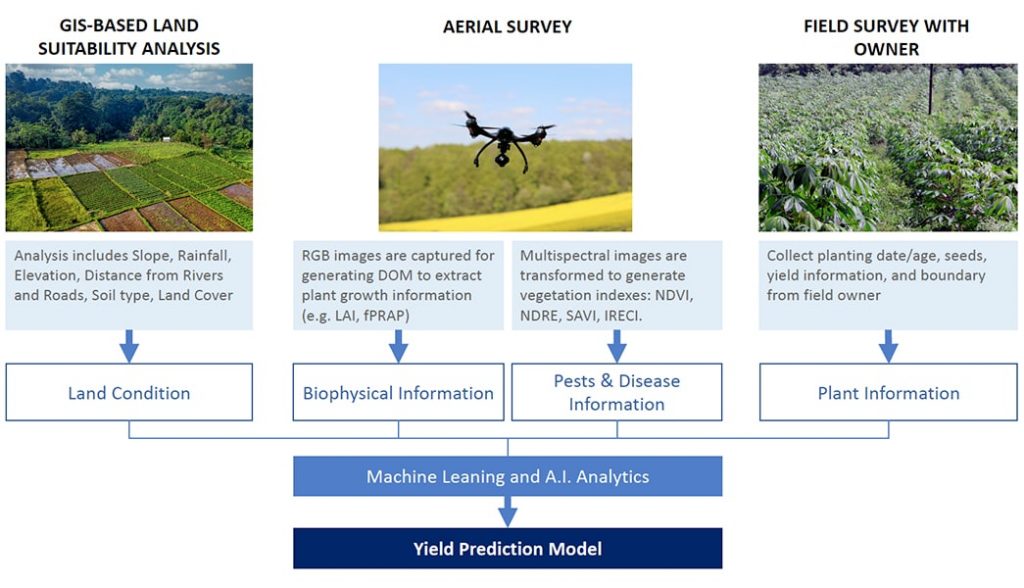
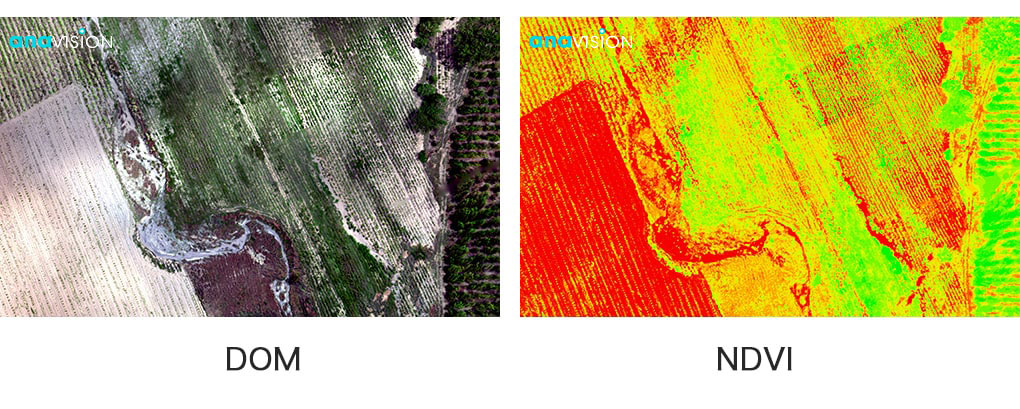
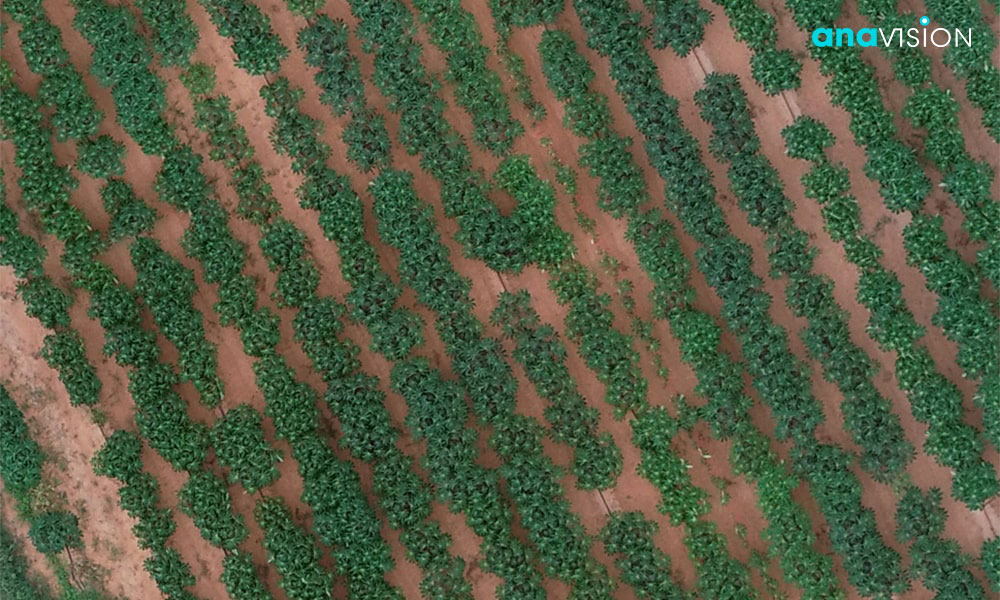
Using AI analytics and machine learning, our team correlates all the information with the captured output of each parameter to continue training the yield prediction model. Once the model is built, it can be used for predicting the yield of the same type of crop in 3 months or 6 months before harvesting.
Anavision strongly believes that remote sensing data has a wide range of applications in the field of agriculture, not limited to cassava, but also can be applied to other crops and plantations such as Oil palm. Captured aerial images can help predict the yield and then to better allocate manufacturer resources for processing the crops. It also can be used in different applications (e.g. weed detection) for precision agriculture.
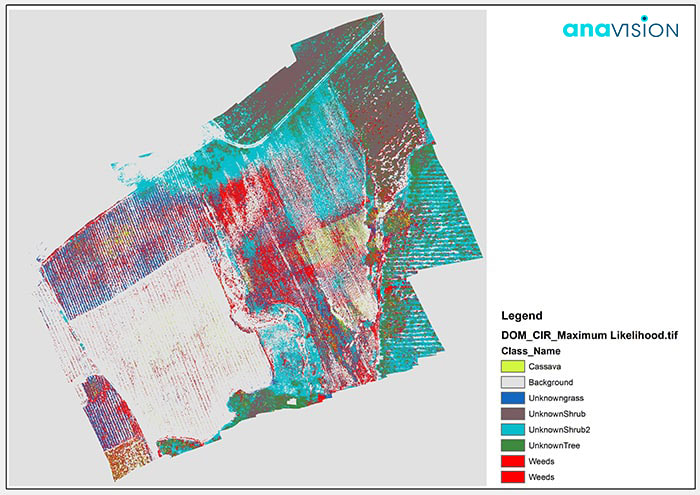
References:
- “Cassava”. Food and Agriculture Organization of the United Nations.
- Fauquet Claude; Fargette Denis (1990). “African Cassava Mosaic Virus: Etiology, Epidemiology, and Control” (PDF). Plant Disease. 74 (6): 404–11. doi:10.1094/pd-74-0404.